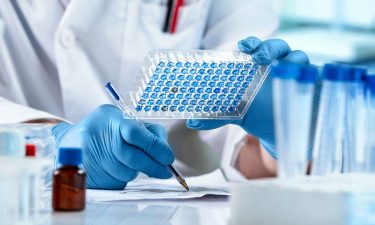
The landscape of scientific and technological advancements has evolved significantly since the finalisation of the first version of ICH Q2 in the 1990s. Particularly notable is the progress in advanced therapies, leading to the emergence of various innovative spectroscopic analytical techniques often utilised as chromatographic detectors (eg, LC‑MS), biological assays and multivariate analytical procedures. However, the absence of guidance for validating these innovative procedures prompted the need for a shift towards Quality by Design (QbD) principles in both regulatory bodies and the pharmaceutical industry.1-4 This transition towards a lifecycle risk‑based approach is reflected in the introduction of ICH guidelines such as Q8-Q14, Q2(R2) and compendial approaches like USP <1220> Analytical Procedure Life Cycle.1-3,5 ICH Q2(R2) integrates principles of knowledge management, while introducing valuable additions for ensuring procedure fitness for use.6
Relationship between ICH Q14 and Q2(R2)
In the new ICH guideline Q14 on Procedure Design,7 emphasis is placed on quality risk management, encouraging the adoption of systematic methodologies including multivariate approaches and modelling to ensure a more comprehensive approach to the procedure lifecycle. Q14 introduces the concept of analytical target profile (ATP) as a technology-independent element, highlighting its vital role in linking the product critical quality attributes (CQAs) from the control strategy with the required performance of the analytical procedure.8 The guideline offers a structured framework for managing post-approval changes and categorising them based on impact and available knowledge, thus supporting greater regulatory flexibility while upholding product quality and safety. Specific guidance for the ongoing monitoring and verification of analytical procedures, however, is lacking. The introduction of “platform analytical procedures” enables streamlined development and validation by utilising existing procedures across multiple products with minimal adjustments.
Extension of scope/techniques in Q2(R2) and the importance of leveraging prior knowledge
Q2(R2) extends validation beyond registration applications to include clinical studies. It broadens applicability to various techniques (eg, UV, IR, NIR, NMR, MS, LC-MS, ELISA, qPCR, etc) and addresses multivariate procedures, helping support the validation of real-time release testing (RTRT). Leveraging prior knowledge from development phases can streamline validation, especially for platform procedures. This approach emphasises efficiency and targets validation efforts effectively, contingent upon industry innovation and control strategy requirements.
Selectivity and specificity
In the latest version, Q2(R2) incorporates the concept of selectivity, acknowledging that specificity is not always possible, extending its use across different techniques while maintaining traditional approaches. Emphasising the importance of demonstrating unbiased measurements, it introduces “technology inherent justification”,6 allowing specificity to be predicted via technical parameters (eg, resolution of isotopes in MS analysis, chemical shifts in NMR, etc), potentially reducing the need for additional experimental studies.
Range and response
In the updated Q2(R2) guidelines, distinctions between “reportable range” and “working range” are clarified, providing precise understanding. While the former pertains to analyte concentration in the sample, derived from specifications and intended use, the latter focuses on analyte concentration in the solution (test concentration). Q2(R2) provides new specific recommendations for:
- assay (eg, new range – 80 percent of lower specification limit to 120 percent of upper specification limit)
- dissolution testing
- purity (as area percentage).6
Furthermore, ICH Q2(R2) outlines three strategies for validating the range: direct assessment of reportable results using calibration models, evaluation of working ranges, and extrapolation when materials with sufficient purity are unavailable. Whether employing univariate methods or multivariate techniques, the response may manifest as linear or non-linear.
Certain procedures, such as immunoassays, cell‑based assays, certain microbiological tests (eg, culture-based assays), and non-linear detectors (eg, evaporative light scattering detectors and charged aerosol detectors for small molecules) exhibit non-linear responses. Various regression methods, encompassing both linear and non‑linear approaches, can be employed to construct the calibration model, where ensuring the model’s predictive accuracy and goodness of fit is paramount to foster confidence in the predicted outcomes (ie, ensuring suitability of calibration model) and assess the range. This highlights the rationale behind replacing “linearity” with “response” in Q2(R1) where a novel section on non-linear response is integrated into Q2(R2). The section “Range” also provides guidance for multivariate procedures. A few aspects of the section’s linear and non-linear response are as follows:
- Linear response: Q2(R2) advances recommendations for evaluating linear response by incorporating residual plot analysis in addition to previous suggestions, such as correlation coefficient (R) or coefficient of determination (R2), and the y-intercept and slope of the regression line.6 This advancement is significant as it addresses the limitations of R2 in estimating linearity, given it is not the ideal metric for assessing the model’s prediction ability, and facilitates the verification of model fit. Notably, Q2(R2) does not discuss single versus multiple points calibration, percentage of area, and weighted linear regression.
- Non-linear response: Q2(R2) highlights the importance of evaluating model suitability through non-linear regression analysis, such as the coefficient of determination.6 While the use of residual plots for non-linear calibration models would have been beneficial, it is absent from this section. Nevertheless, in non-linear analysis, examining residual characteristics remains important, similar to linear models, with relevant assumptions such as normality, independence and homoscedasticity. Importantly, estimating detection or quantification limits via signal‑to‑noise ratio extrapolation from a single high-level standard, common with linear detectors, is unsuitable for non-linear detectors and can lead to misleading results.
In Q2(R2), “detection limit and quantitation limit” are now termed “lower range limit,” while traditional assessment methods are supplemented by a new impurity testing criterion: the lower range limit must meet or fall below the reporting threshold. Additionally, if the lower range limit substantially exceeds the reporting limit (eg, by 10-fold), justifications may support omitting confirmatory evaluation.6
Accuracy and precision: independent and combined assessment
While keeping the independent assessment as in the previous guideline version, ICH Q2(R2) expands the recommendation for how to assess accuracy (bias) and precision in combination.8 Accuracy reporting involves presenting the mean percent recovery, or the difference between the mean and the accepted true value, along with an appropriate confidence interval.6 Precision is reported using standard deviation, relative standard deviation and suitable confidence intervals.6 ICH Q2(R2) does not discuss precision of the reportable result or optimisation through replication.9-10
Despite providing examples for several techniques, some areas lack coverage, such as bioassay examples, and lack key aspects such as recommended acceptance criteria and residual plots
Overall, Q2(R2) covers the importance of uncertainty by setting the expectation for the estimation of suitable confidence intervals (or justified alternative statistical intervals), which should be compatible with the acceptance criteria (unless otherwise justified) for accuracy and precision assessment, representing a new addition compared to Q2(R1). However, it lacks clarity on whether the need for such assessments is universally expected or contingent upon risk assessment – low-risk cases may not require this assessment. Logically, the interval testing could require a larger number of validation experiments to generate data and be compatible with reasonable acceptance limit. Furthermore, Q2(R2) refers to a new alternative combined approach for assessing accuracy (bias) and precision, which may involve estimating prediction, tolerance or confidence intervals and comparing them to relevant performance criteria,6 although the guideline does not offer much guidance beyond this mention. USP <1210> Statistical Tools for Procedure Validation offers guidance for estimating confidence, prediction and tolerance intervals for independent and combined assessment.11 Q2(R2) covers accuracy and precision assessment for multivariate procedures, including comparing root mean square error of prediction (RMSEP) to root mean square error of calibration (RMSEC) for quantitative methods and using classification/misclassification rate or positive prediction rate for qualitative methods. Additionally, USP <1039> Chemometrics12 is under review to offer a framework for ensuring procedure suitability throughout the multivariate procedure lifecycle.
Conclusion
The revised ICH Q2(R2) guideline updates terminology and broadens the scope of techniques/products applicable, improving validation requirements for uni- and multivariate analytical procedures, while maintaining traditional approaches.
While new sections and concepts like non‑linear response and residual plots enhance clarity, knowledge gaps remain, highlighting the need for additional guidance.
ICH Q2(R2) could enhance its coverage to address certain aspects such as residual plot importance for non-linear models and specific guidance for linear response methods (eg, single vs multiple points calibration, percentage of area, and weighted linear regression).
Despite providing examples for several techniques, some areas lack coverage, such as bioassay examples, and lack key aspects such as recommended acceptance criteria and residual plots.
While Q2(R2) improves in ensuring procedure fitness for use, confusion may arise, particularly regarding the replication design for establishing the reportable value and for validation studies (section 2.1), where one may understand the validation study should be conducted with the same number of replicates used in routine analysis, which is not necessarily always the case. The reportable value derives from sample analysis using defined replication strategy for specification comparison, while validation aims to demonstrate procedure performance adequacy.
Although Q2(R2) places consideration on uncertainty by recommending the reporting of suitable intervals for independent accuracy and precision assessment (and in combination), further guidance is needed to report the confidence intervals and the impact of number of determinations for the combined approach. Additionally, Q2(R2) lacks elements like the ATP, an area where alignment with Q14 could be improved.
Q14 lacks guidance on ongoing monitoring, which is addressed in USP publications.5,13 Q2(R2) briefly touches on utilising prior knowledge from the development stage and potential omission of validation tests based on science- and risk‑based justifications. However, further guidance on these matters and a clearer alignment with Q14 activities are lacking. This gap can be addressed by referring to USP chapter <1220>, which emphasises the importance of defining a suitable replication strategy to reduce the reportable value uncertainty. Overall, while both Q14 and Q2(R2) align with principles outlined in USP <1220>, further clarity and alignment between the two guidelines are needed to promote a comprehensive lifecycle approach.
About the authors
Amanda Guiraldelli Mahr
Amanda is Scientific Affairs Manager at USP and serves as the scientific liaison for the USP Analytical Procedure Lifecycle JS. She holds a bachelor’s degree in Pharmacy Biochemistry and a PhD in Analytical Chemistry from the University of São Paulo.
Joachim Ermer
Joachim is a member of the USP Analytical Procedure Lifecycle JS and holds a master’s degree and PhD in Biochemistry from the University of Halle-Wittenberg, Germany.
Phil Borman
Phil is a member of the USP Analytical Procedure Lifecycle JS and holds a master’s degree in Analytical Chemistry from the University of Manchester, a master’s degree in Applied Statistics from De Montfort University and a doctorate of science degree from the Leicester School of Pharmacy.
Jaime Marach
Jaime is a member of the USP Analytical Procedure Lifecycle JS and holds a bachelor’s degree in Biochemistry from California State University, Northridge and a PhD in Biomedical Sciences from the University of California, San Diego.
Pierre Lebrun
Pierre is a member of the USP Analytical Procedure Lifecycle JS and holds a bachelor’s degree in Computer Sciences and in Statistics from UCLouvain, Belgium, and a PhD in Sciences (Statistics) from the University of Liège, Belgium. He is a scientific collaborator and lecturer at the University of Liège.
Jane Weitzel
Jane is a member of the USP Analytical Procedure Lifecycle Joint Subcommittee (JS) and holds a bachelor’s degree in Chemistry from University of Manitoba, Canada.
Jean-Marc Roussel
Jean-Marc is a member of the USP Analytical Procedure Lifecycle JS and holds a master’s degree and a PhD in Analytical Chemistry from the University of Aix-Marseille III, France.
Christopher Burgess
Christopher is a member of the USP Analytical Procedure Lifecycle JS and holds a master’s degree and a PhD in Analytical Chemistry from the University of Loughborough.
References
1. Guiraldelli A, Weitzel J. Evolution of Analytical Procedure Validation Concepts: Part I – Analytical Procedure Life Cycle and Compendial Approaches. Pharmaceutical Technology. 2022.
2. Guiraldelli A, Weitzel J. Evolution of Analytical Procedure Validation Concepts: Part II– Incorporation of Science and Risk-based Principles in ICH Q14 and Q2(R2) Guidelines. Pharmaceutical Technology. 2022.
3. Teasdale A, Borman PJ, Mullen AK. Regulatory Highlights. Org Process Res Dev. 2022 Apr 15;26(4):1029–37.
4. Borman P, Chatfield M, Nethercote P, et al. The Application of Quality by Design to Analytical Methods. Pharmaceutical Technology. 2007;31(10):142–52.
5. USP General Chapter <1220> Analytical Procedure Lifecycle. 2022.
6. ICH Guideline Q2(R2) Validation of Analytical Procedures. 2022.
7. ICH Guideline Q14 Analytical Procedure Development. 2023.
8. Jackson P, Borman P, Campa C, et al. Using the Analytical Target Profile to Drive the Analytical Method Lifecycle. Anal Chem. 2019; 19;91(4):2577-2585.
9. Borman P, Schofield T, Lansky D. Reducing uncertainty of an analytical method through efficient use of replication. Pharmaceutical Technology. 2021;45(4):48–56.
10. Ermer J, Agut C. Precision of the reportable result. Simultaneous optimisation of number of preparations and injections for sample and reference standard in quantitative liquid chromatography. J Chromatogr A. 2014; (1)1353:71–7.
11. USP General Chapter <1210> Statistical Tools for procedure Validation.
12. USP General Chapter <1039> Chemometrics. USP-NF [Internet]. Available from: https://doi.org/10.31003/USPNF_M2345_02_01
13. Borman PJ, Guiraldelli AM, Weitzel J, et al. Ongoing Analytical Procedure Performance Verification Using a Risk-Based Approach to Determine Performance Monitoring Requirements. Analytical Chemistry. 2024 Jan 8;96(3):966–79. doi:10.1021/acs.analchem.3c03708
The post Advancements and knowledge gaps in ICH Q2(R2) appeared first on European Pharmaceutical Review.